How do you segment based on Customer Lifetime Value (CLV)?
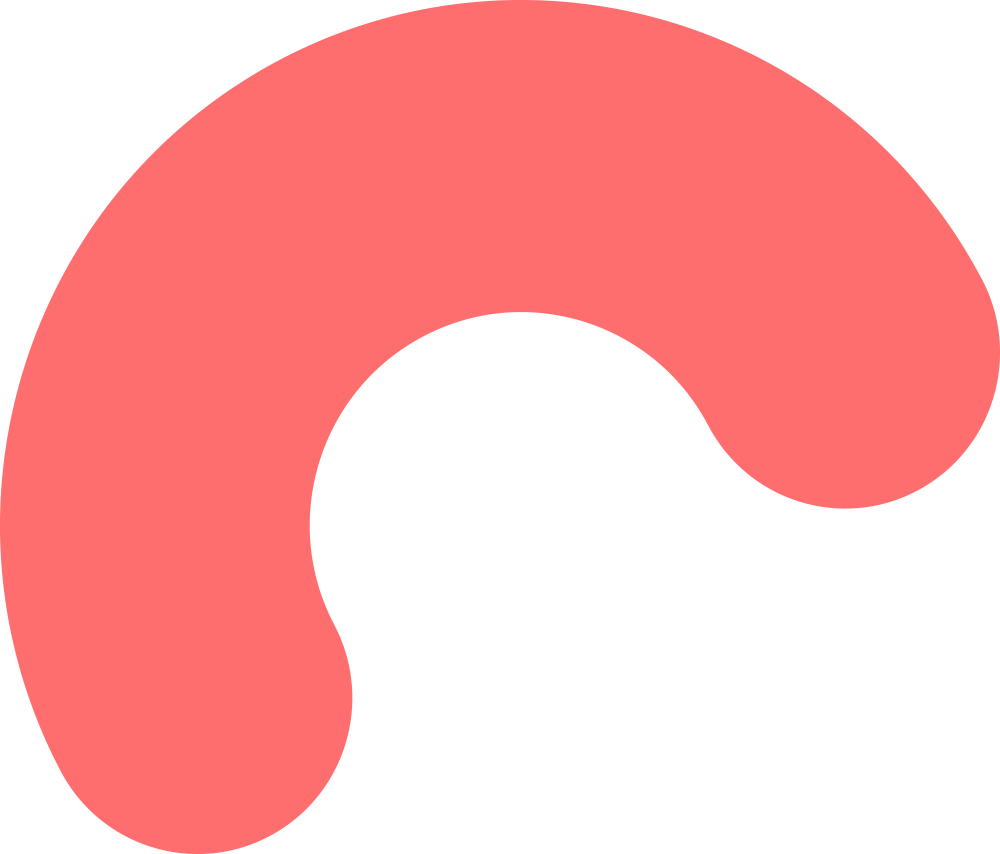
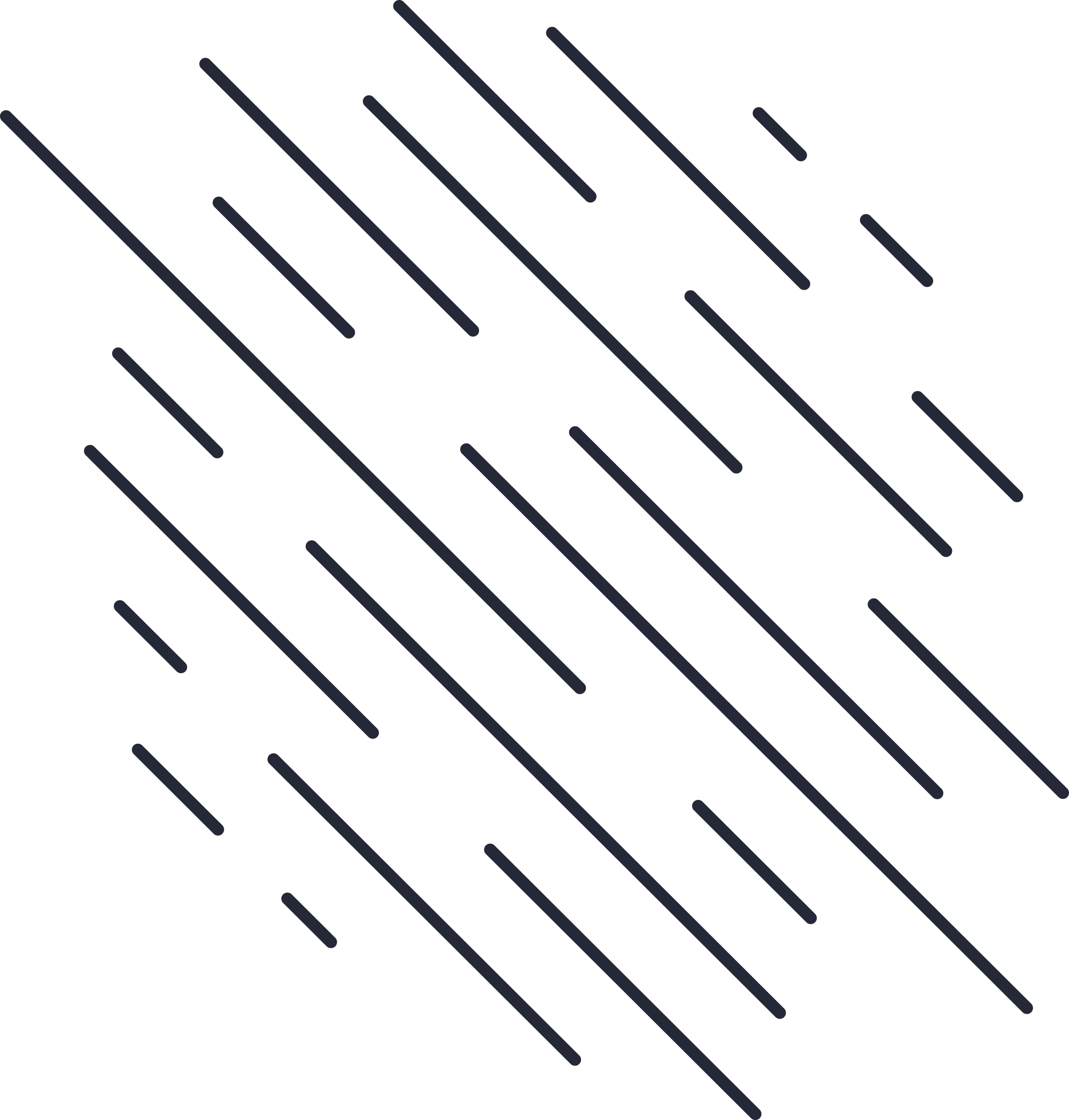
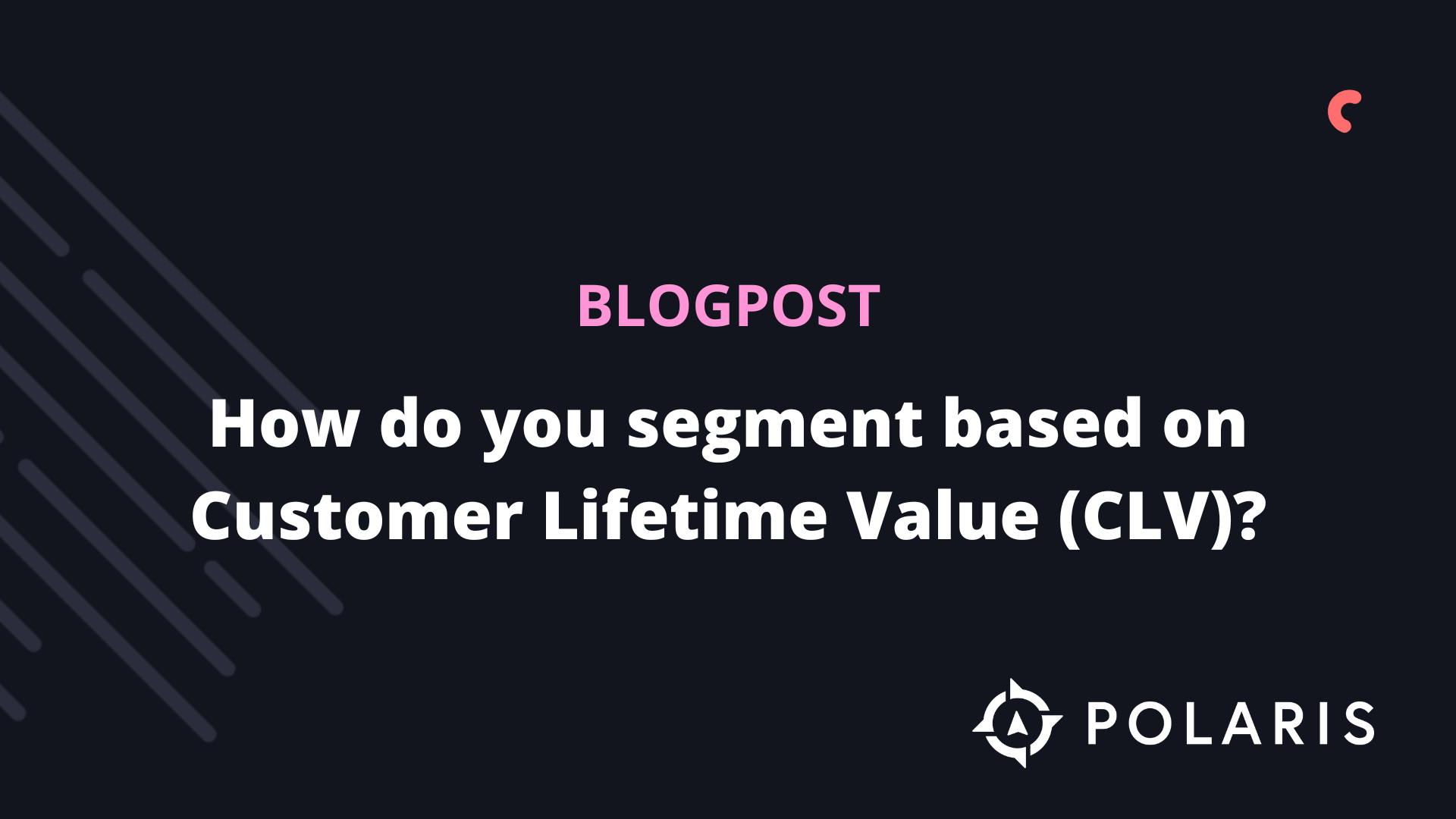
Ever wondered how to create a Customer Lifetime Value (CLV) segment? And are you curious about how to export all the important CLV data? In this article, we’ll explain everything you need to know.
Customer Lifetime Value
CLV is part of Klaviyo’s predictive analytics and is a powerful tool for segmentation. Customer Lifetime Value represents the total amount (both ‘historic’ and ‘predictive’) that a customer spends on your brand over time. With CLV segments, you can group customers based on this value, allowing you to send relevant content and trigger segment-based flows. For example, you can use historic CLV to build a VIP welcome flow or use predictive CLV to send targeted campaigns to customers predicted to spend a certain amount within a year.
Before You Start
Before creating a CLV segment, it’s important to know that you can only segment by CLV if:
- At least 500 customers have placed an order. This refers to people who have actually made a purchase in your store, not just active profiles. If a profile is active but has no purchase data, Klaviyo won’t have enough information to make a prediction;
- You have an e-commerce integration (e.g., Shopify, BigCommerce, or Magento) or use Klaviyo’s API to send placed orders;
- You have at least 180 days of order history and have received orders in the past 30 days;
- You have some customers who have placed 3 or more orders.
Creating a CLV Segment
To create a segment based on available CLV properties (i.e., ‘historic’, ‘predictive’, and ‘total’ CLV), use the ‘predictive analytics about someone’ condition. Then, select the desired metric and value. See the image below.

Example
Let’s say the average order value for your customers is €15. You might want to target customers who don’t meet this average order value with discounts to encourage another purchase.
To achieve this, you can create a segment of customers who are predicted to spend no more than €5. You can target these customers with a discount campaign, similar to a winback or re-engagement campaign. If you’re targeting through email, you should include the following conditions:
- The customers belong to your main email list (in this case, a newsletter list);
- They’ve opened an email within a certain period. Adding this condition ensures that you’re sending emails to engaged subscribers. In the example below, the time frame is the last 90 days.
By setting up these conditions, you can effectively target the right customers for your campaign.
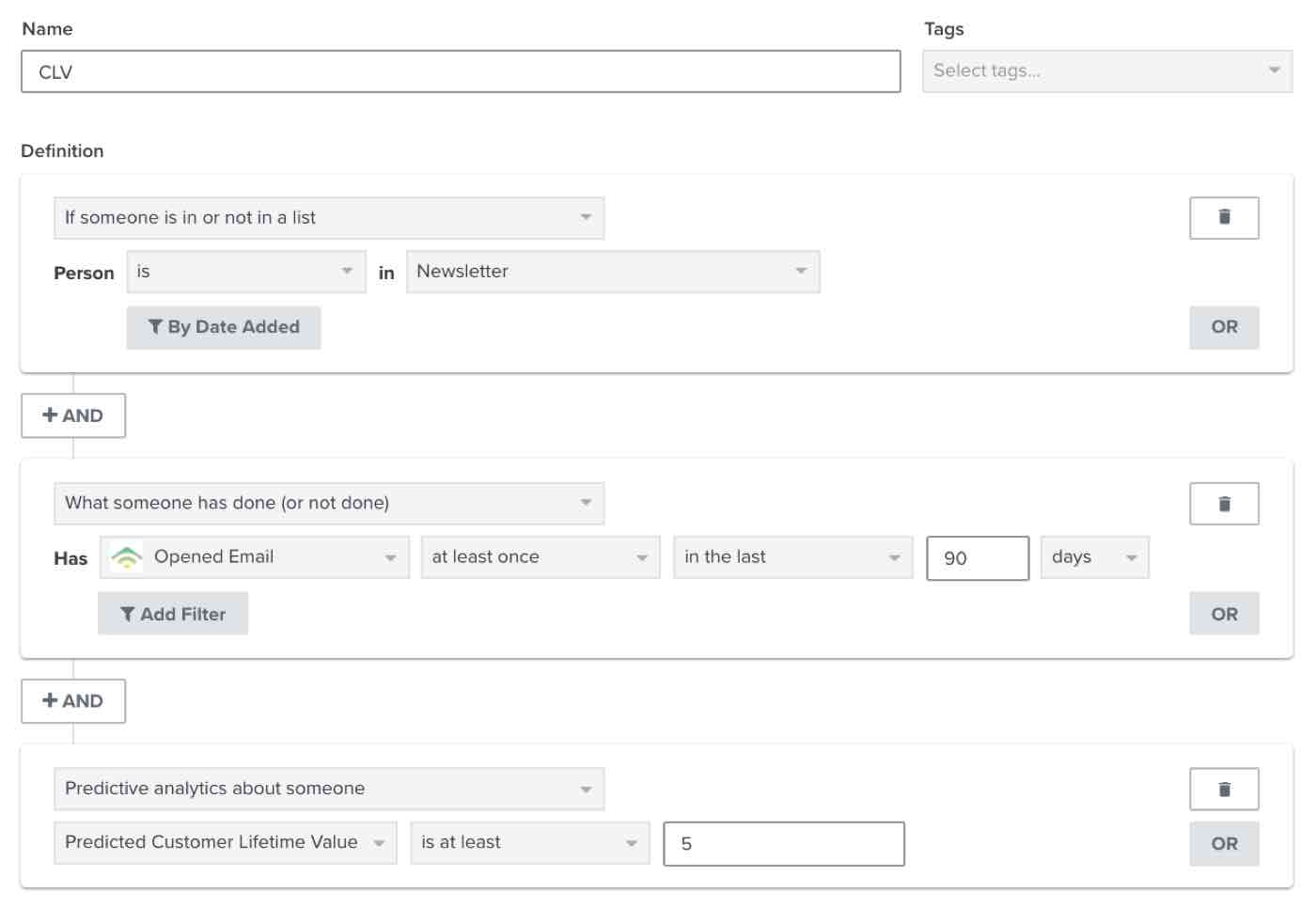
Export CLV Segments
By exporting CLV data, you can further analyze and predict the behavior of different customer groups. In addition to your CLV and predictive analyses, you can also export your Churn Risk Prediction. The churn risk is exported to your CSV file as a number between 0 and 1. For example, a number like 0.45 would correspond to a 45% churn risk.
This data allows for deeper insights into customer behavior, helping you to strategize and improve customer retention efforts.
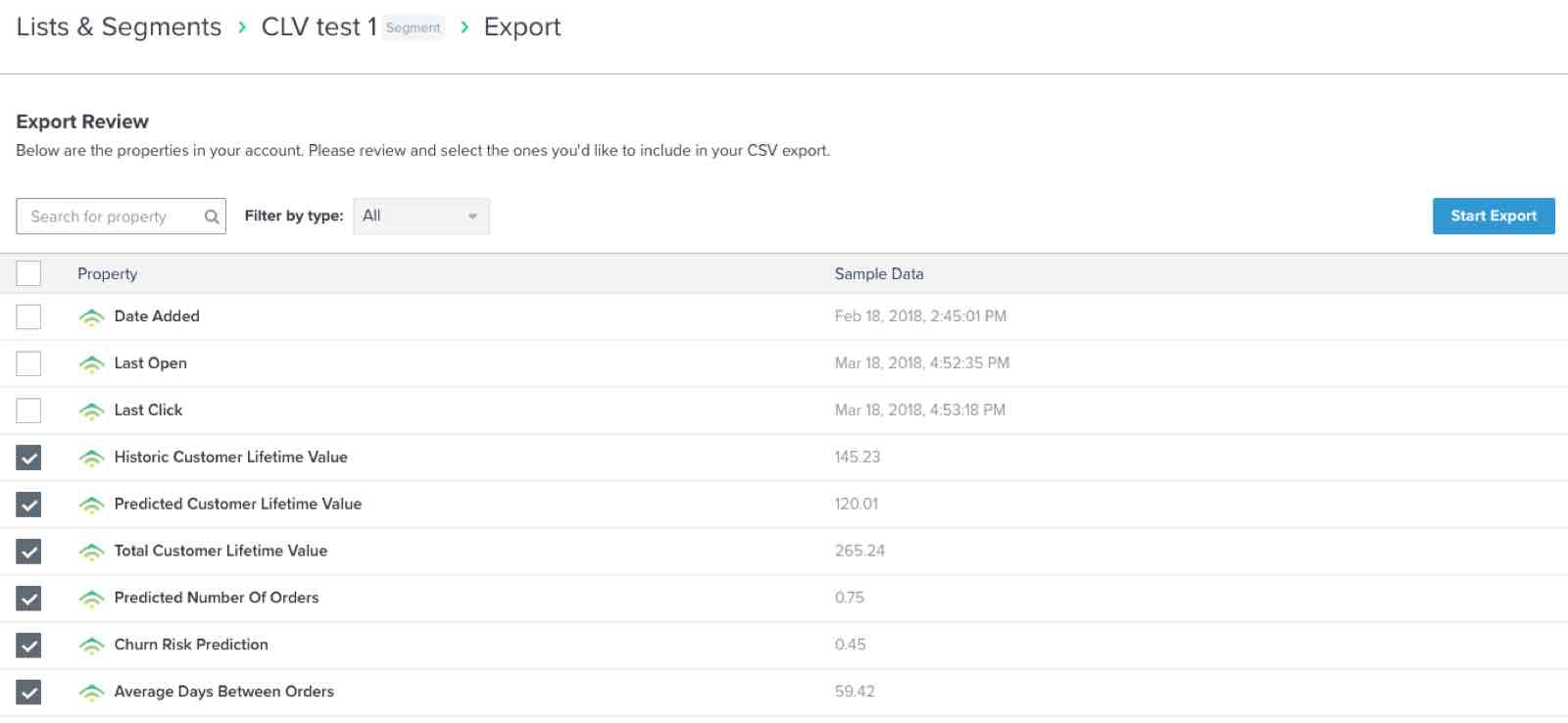
If you have many one-time buyers, you likely have a high average churn risk. To reduce your average churn risk, you can focus your marketing efforts on retaining customers after their first purchase. Use Klaviyo’s Predicted CLV metric to identify people who are unlikely to make another purchase, similar to the example above.
Once you export this data as a CSV, you can perform your own analyses. Here are some useful calculations:
- Average CLV: Calculate the average customer value of a segment by taking the average of both the historic CLV and total CLV.
- Predict future spending for a segment: Add up the predictive CLV of all members in a segment to get the expected revenue from customers in this segment for the next year.
- Estimate the number of returning customers: First, calculate the average Churn Risk Prediction values. Then, subtract the average from 1. Multiply the result by the number of people in the segment. This gives you an estimate of the number of customers expected to return.
Want to learn more about Customer Lifetime Value (CLV)? Check out this blogpost on CLV for e-commerce
Find the original article here.